Machine Translation for the Localization Use Case
Overview of requirements for successful MT use in Localization and why it is the most difficult use case for MT
We live in an era where MT is translating more than 99% of all the translation being done on the planet on any given day. However, the adoption of MT by the enterprise is still nascent and still building momentum.
Business enterprises have been slower to adopt MT even though national security and global surveillance-focused government agencies have used MT heavily. This adoption delay has mostly been because MT has to be adapted and tuned to perform better with very specific language used in specialized enterprise content.
Early enterprise adoption of MT was focused on eCommerce and customer support use-cases (IT, Auto, Aerospace) where huge volumes of technical support content made it a necessity to use MT technology to allow any possibility of translating the voluminous content in a timely and cost-effective manner to improve the global customer experience.
Microsoft was a pioneer who translated its widely used technical knowledge base to support an increasingly global customer base. The positive CX feedback they received for doing this from around the world, led to many other large IT and consumer electronics firms following.
The adaptation of the MT system to perform better on enterprise content is a critical requirement in producing successful outcomes.
In most of these early use-cases we see that MT is used to manage translation challenges when the content volumes were huge, i.e., millions of words a day or week. These were “either use MT or provide nothing” knowledge-sharing scenarios.
These enterprise-optimized MT systems have to adapt to the special terminology and linguistic style of the content they translate, and this customization has been a key element of success with any enterprise use of MT.
eBay was an early MT adopter in eCommerce and has stated often that MT is key in promoting cross-border trade. It was understood that “Machine translation can connect global customers, enabling on-demand translation of messages and other communications between sellers and buyers, and helps them solve problems and have the best possible experiences on eBay.”
A study by an MIT economist showed that after eBay improved its automatic translation program in 2014, commerce shot up by 10.9 percent among pairs of countries where people could use the new system.
Today we see that MT is a critical element of the global strategy for Alibaba, Amazon, eBay, and many other eCommerce giants.
Even in the COVID-ravaged travel market segment, MT is critical as we see with Airbnb, which now translates billions of words a month to enhance the international customer experience on their platform. In November 2021 Airbnb announced a major update to the translation capabilities of their platform in response to rapidly growing cross-border bookings and increasing international WFH activity.
“The real challenge of global strategy isn’t how big you can get, but how small you can get.”
Dennis Goedegebuure, former head of Global SEO at Airbnb.
However, MT use for localization use cases has trailed far behind these leading-edge examples, and even in 2021, we find that the adoption and active use of MT by Language Service Providers (LSPs) is still low. Much of the reason lies in the fact that LSPs work on hundreds or thousands of small projects rather than a few very large ones.
Early MT adopters tend to focus on large-volume projects to justify the investments needed to build adapted systems capable of handling the high-volume translation challenge.
What options may be available to increase adoption in the localization and professional business translation sectors?
At the MT Summit conference in August 2021, CSA analyst Arle Lommel shared survey data on MT use in the localization sector in his keynote presentation. He noted that while there has been an ongoing increase in adoption by LSPs there is considerable room to grow.
Arle specifically pointed out that a large number of LSPs who currently have MT capacity only use it for less than 15% of their customer workload and, “our survey reveals that LSPs, in general, process less than one-quarter of their [total] volume with MT.”
The CSA survey polled a cross-section of 170 LSPs (from their "Ranked 191" set of largest global LSPs) on their MT use and MT-related challenges. The quality of the sample is high and thus these findings are compelling.
The graphic below highlights the survey findings.
CSA Survey of MT Use at LSPs
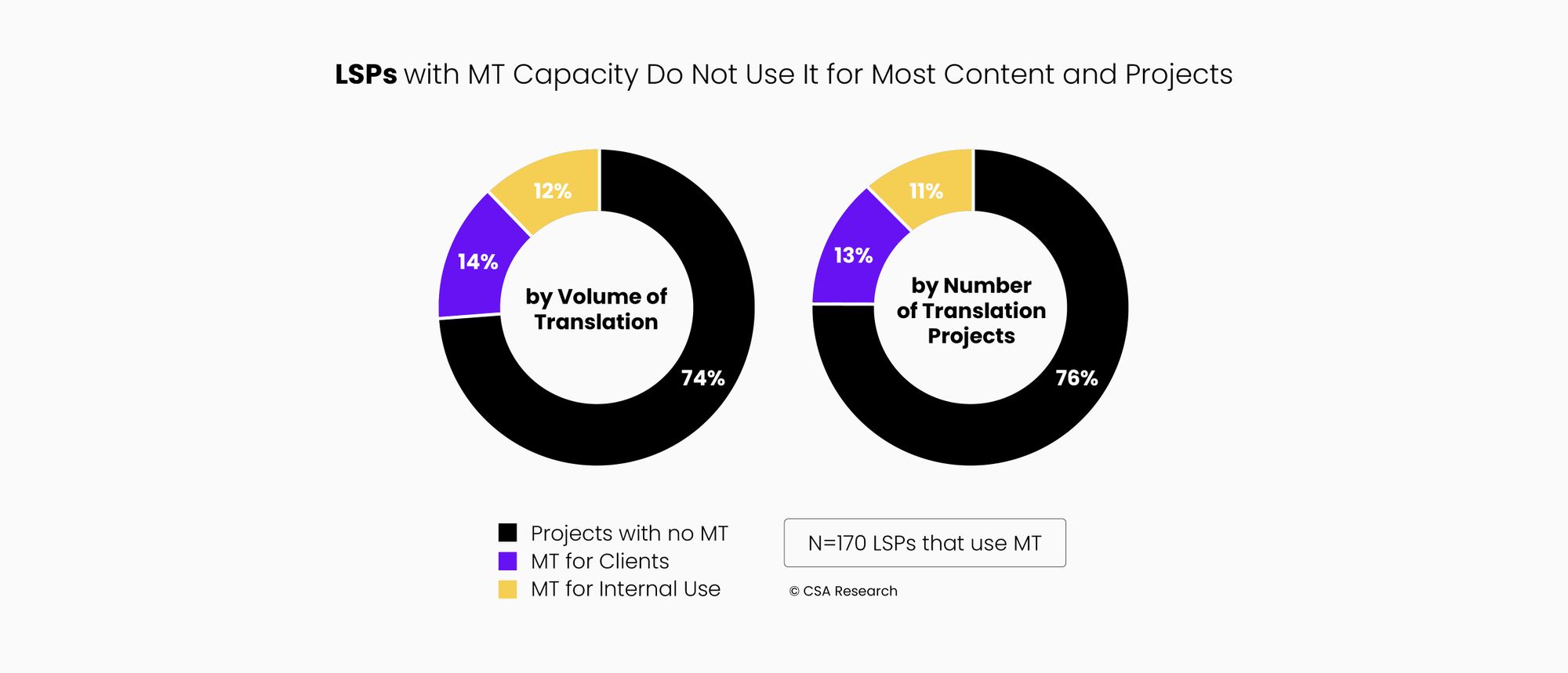
After probing further into the reasons behind the relatively low use of MT in the LSP sector they discovered the following:
- 72% of LSPs report difficulty in meeting quality expectations with MT
- 62% of LSPs struggle with estimating effort and cost with MT
Both of these causes point to the difficulty that most LSPs face with the predictability of outcomes with an MT project.
Arle reported that in addition to LSPs, many enterprises also struggle with meeting quality expectations and are often under pressure to use MT in inappropriate situations or face unrealistic ROI expectations from management. Thus, CSA concluded that while current-generation MT does well relative to historical practice, it does not (yet) consistently meet stakeholder requirements.
This apparent market reality validated by this representative sample is in stark contrast to what happens at Translated Srl, where 95% of all projects and all client work use MT (ModernMT) since it is a proven way to expedite and accelerate translation productivity.
Adaptive, continuously learning ModernMT has been proven to work effectively over thousands of projects with tens of thousands of translators.
This ability to properly use MT in an effective and efficient assistive role in production translation work has resulted in Translated being one of the most efficient LSPs in the industry, with the highest revenue per employee and high margins.
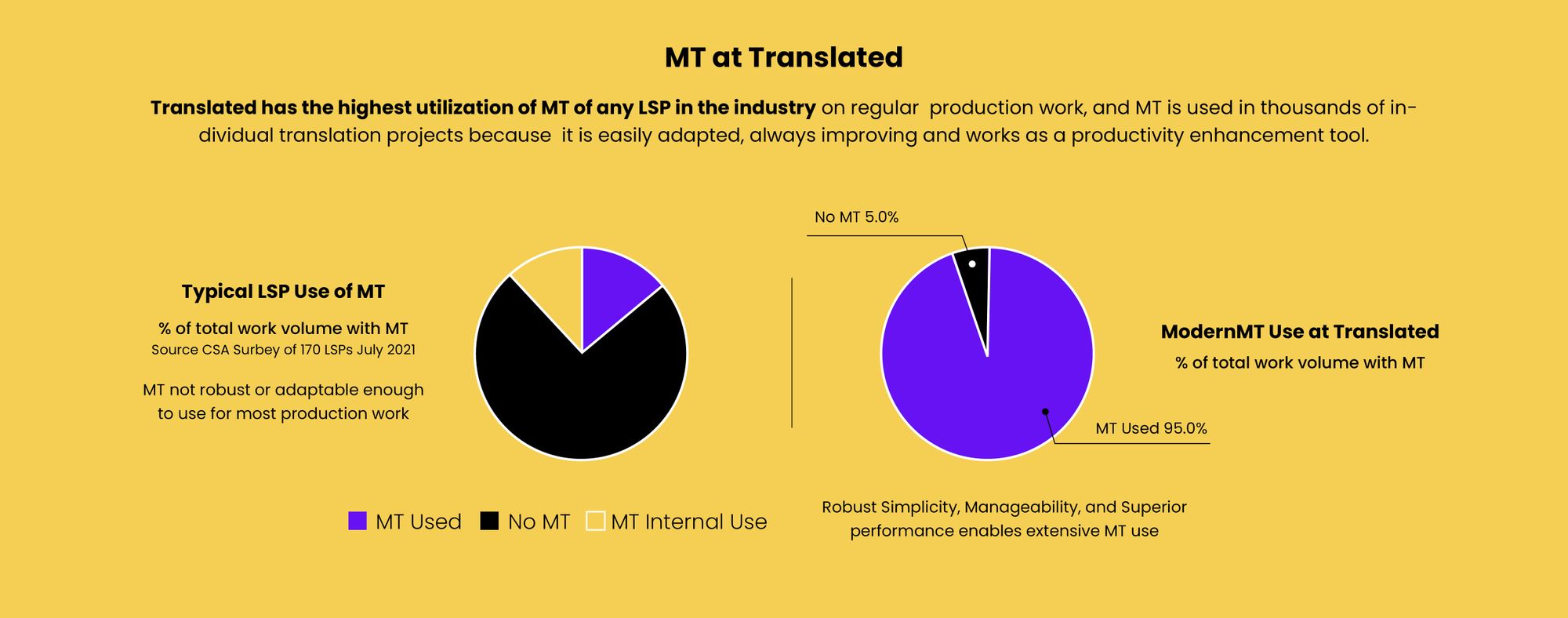
Another example of the typical LSP experience: a recent study by Charles University done with only 30 translators using 13 engines (EN>CS) concludes: "the previously assumed link between MT quality and post-editing time is weak and not straightforward." They also found that these translators had “a clear preference for using even imprecise TM matches (85–94%) over MT output."
This is hardly surprising, as getting MT to work effectively in production scenarios requires more than choosing the system with the best BLEU score.
Understanding The Localization Use Case For MT
Why is MT so difficult for LSPs to deploy in a consistently effective and efficient manner?
There are at least four primary reasons:
- The localization use case requires the highest quality MT output to drive productivity which is only possible with specialized expertise and effort,
- Most LSPs work on hundreds/thousands of smallish projects (relative to MT scale) that can vary greatly in scope and focus,
- Effective MT adaptation is complex,
- MT system development skills are not typically found in an LSP team.
MT Output Expectations
As the CSA survey showed, getting MT to consistently produce output quality to enable use in production work is difficult. While using generic MT is quite straightforward, most LSPs have discovered that rapidly adapting and optimizing MT for production use is extremely difficult.
It is a matter of both MT system development competence and workflow/process efficiency. Many LSPs feel that success requires the development of multiple engines for multiple domains for each client, which is challenging since they don't have a clear sense of the effort and cost needed to achieve positive ROI.
If you don’t know how good your MT output will be, how do you plan for staffing PEMT work and calculate PEMT costs?
Thus, we see MT is only used when very large volumes of content are focused around a single subject domain or when a client demands it.
A corollary to this is that it requires deep expertise and understanding of NMT models to acquire the skills and data needed to raise production MT output to useful high-quality levels consistently.
Project Variety & Focus
Most LSPs handle a large and varied range of projects that cover many subject domains, content types, and user groups on an ongoing basis. The translation industry has evolved around a Translate>Edit>Proof (TEP) model that has multiple tiers of human interaction and evaluation in a workflow.
Most LSPs struggle to adapt this historical people-intensive approach to an effective PEMT model which requires a deeper understanding of the interactions between data, process, and technology.
The biggest roadblock I have seen is that many LSPs get entangled in opaque linguistic quality assessment and estimation exercises, which completely misses the business value created by making more content available. Localization is only one of several use-cases where translation can add value to the global enterprise's mission.
Typically, there is not enough revenue concentration around individual client subject domains, thus, it is difficult for LSPs to invest in building MT systems that would quickly add productivity to client projects. MT development is considered a long-term investment that can take years to yield consistently positive returns.
This perceived requirement for the development of multiple engines for many domains for each client requires an investment that cannot be justified with short-term revenue potential. MT projects, in general, need a higher level of comfort with uncertainty and handling hundreds of MT projects concurrently to service the business is too demanding a requirement for most LSPs.
MT is Complex
Many LSPs have dabbled with open-source MT (Moses, OpenNMT) or AutoML and Microsoft Translator Hub only to find that everything from data preparation to model tuning, and quality measurement is complicated and requires deep expertise that is uncommon in the language industry.
While it is not difficult to get a rudimentary MT model built, it is a very different matter to produce an MT engine that consistently works in production use. For most LSPs, open-source and DIY MT is the path to a failed project graveyard.
Neural MT technology evolution is happening at a significantly faster pace than Statistical MT. To stay abreast with the state-of-the-art (SOTA) requires a serious commitment, both in manpower and computing resources.
LSPs are familiar with translation memory technology that has barely changed in 25 years, but MT has changed dramatically over the same period. In recent years the neural network-based revolution has driven multiple open-source platforms to the forefront and keeping abreast with the change is difficult.
NMT requires expertise not only around "big data" and algorithms and open-source platform alternatives but also around understanding parallel processing hardware.
Today AI and Machine Learning (ML) are synonymous, and engineers with ML expertise are in high demand.
MT requires long-term commitment and investment before consistent positive ROI is available and few LSPs have an appetite for such investments.
Some say that an MT team might be ready for prime-time production work only after they have built a thousand engines and have this experience to draw from. This competence-building experience seems to be a requirement for sustainable success.
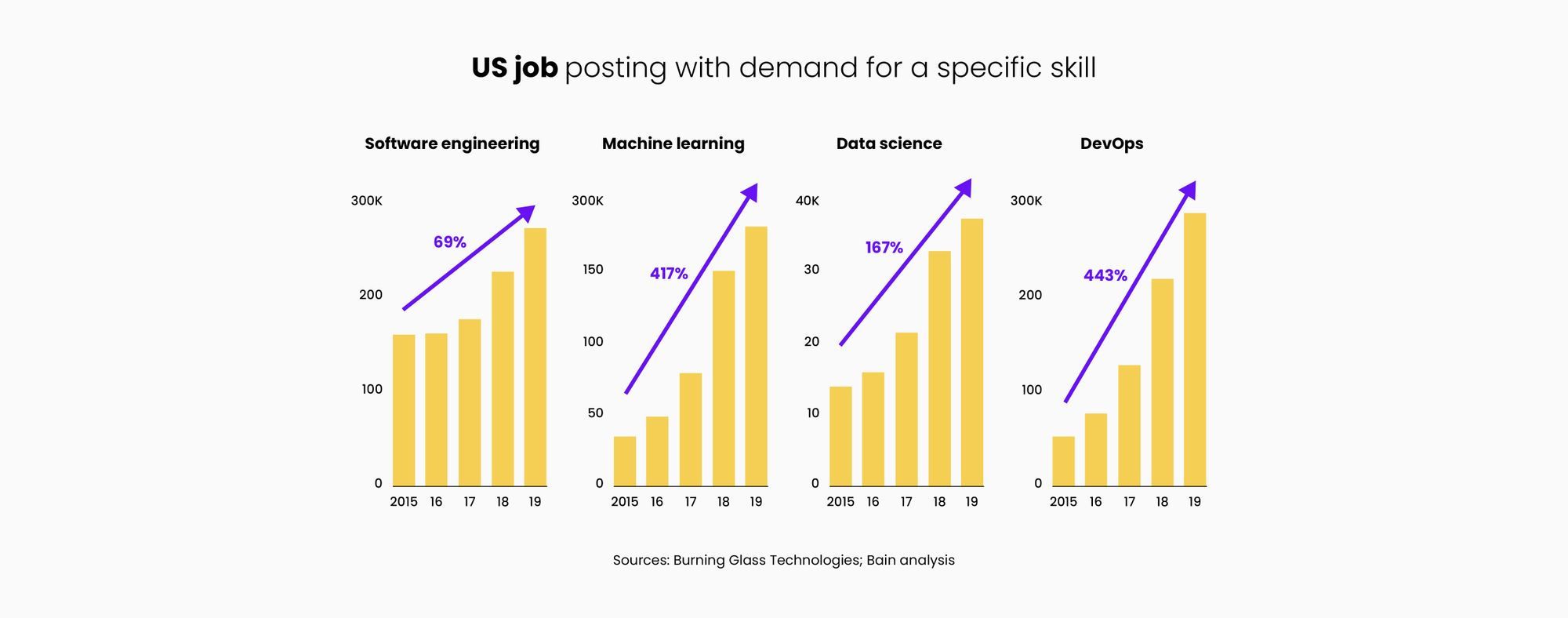
Talent Shortage
Even if LSP executives are willing to make these strategic long-term investments, finding the right people has gotten increasingly harder. According to a recent survey by Gartner, executives see the talent shortage not just as a major hurdle to progressing organizational goals and business objectives, but it is also preventing many companies from adopting emerging technologies.
The Gartner research, which is built on a peer-based view of the adoption plans of 111 emerging technologies from 437 IT global organizations over a 12- to 24-month time period, shows that talent shortage is the most significant adoption barrier to 64% of emerging technologies, compared with just 4% in 2020.
IT executives cited talent availability as the main adoption risk factor for the majority of IT automation technologies (75%) and nearly half of digital workplace technologies (41%).
But using technology early and effectively creates competitive advantage. Bain estimates that “born-tech” companies have captured 54% of the total market growth since 2015. “Born-tech” companies are those with a tech-led strategy. Think Tesla in automobiles, Netflix in media, and Amazon in retail.
Technology has emerged as the primary disruptor and value creator across all sectors. The demand for data scientists and machine learning engineers is at an all-time high.
LSPs need to compete with the global 2000 enterprises who offer more money and resources to the same scarce talent. Thus, we even see technical talent migrating out of translation services to the “mainstream” industry.
There is a gold rush happening around well-funded ML-driven startups and enterprise AI initiatives. These skills are being seen as critical to the next major evolution in value creation in the overall economy as the chart below shows.
This perception is driving huge demand for data scientists, ML engineers, and computational linguists who are all necessary to build momentum and produce successful AI project outcomes. The talent shortage will only get worse as more people realize that deep learning technology is fueling most of the value growth across the global economy.
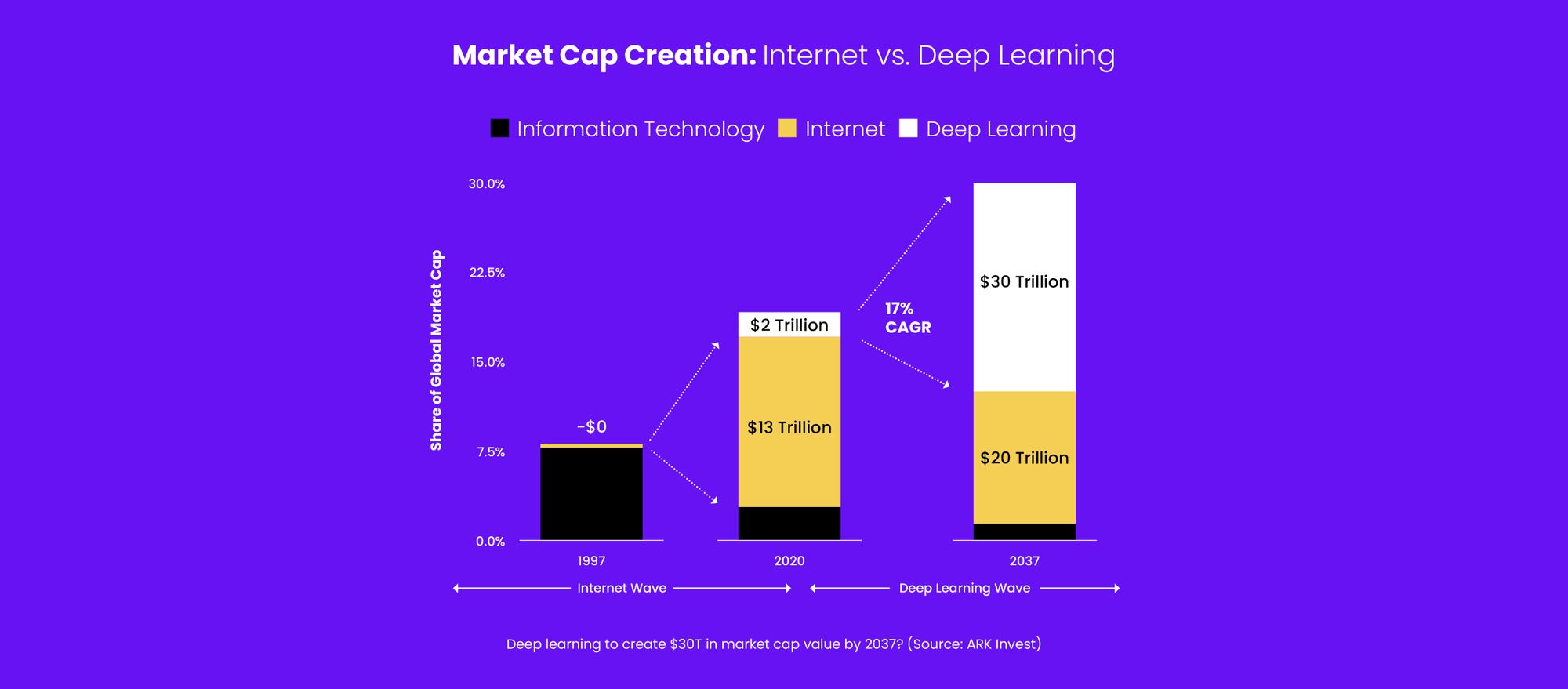
Thus, it appears that MT is likely to remain an insurmountable challenge for most LSPs. The option for an LSP to start building robust state-of-the-art MT capabilities in 2021 is increasingly unlikely. Even the largest LSPs today have to use “best-of-breed” public systems rather than build internal MT competence. Strategies employed to do this typically depend on selecting MT systems based on BLEU, hLepor, TER, Edit Distance, or some other score-of-the-day, which again explains why there is <15% MT-in-production-use.
As CSA has discovered, this effort has been largely unsuccessful because a good Edit Distance/hLepor/Comet score does not necessarily translate to responsiveness, ease of use, adaptability of the MT system to the production localization use-case needs.
For MT to be useable on 95%+ of the production translation work done by an LSP, it needs to be reliable, flexible, manageable, rapidly adaptive, and continuously learning. MT needs to produce predictably useful output and be truly assistive technology for it to work in localization production work.
The contrast of the MT experience at Translated Srl is striking. ModernMT was designed from the outset to be useful to translators and created to collect the right kind of data needed to rapidly improve and assist in localization project-focused systems.
ModernMT is a blend of the right data, deep expertise in both localization processes and machine learning, and a respectful and collaborative relationship between translators and MT technologists. It is more than just an adaptive MT engine.
Translated has been able to overcome all of the challenges listed above using ModernMT, which today is possibly the only viable MT technology solution that is optimized for the core localization-focused business of LSPs.
ModernMT is the creation of an MT system optimized for generic LSP use. It could be used quickly and successfully by any LSP as there is no startup setup and training needed, it is a simple "load TM and immediately use" model.
ModernMT Overview & Suitability for Localization
ModernMT is an MT system that is responsive, adaptable, and manageable in the typical localization production work scenario. It is an MT system architecture that is optimized for the most demanding MT use-case: localization. And it is thus able to handle many other use-cases which may have more volume but are less demanding on the output quality requirements.
ModernMT is a context-aware, incremental, and responsive general-purpose MT technology that is price competitive to the big MT portals (Google, Microsoft, Amazon) and is uniquely optimized for LSPs and any translation service provider, including individual translators.
It can be kept completely secure and private for those willing to make the hardware investments for an on-premise installation. It is also possible to develop a secure and private cloud instance for those who wish to avoid making hardware investments.
ModernMT overcomes technology barriers that hinder the wider adoption of currently available MT software by enterprise users and language service providers:
- ModernMT is a ready-to-run application that does not require any initial training phase. It incorporates user-supplied resources immediately without needing upfront model training.
- ModernMT learns continuously and instantly from user feedback and corrections made to MT output as production work is being done. It produces output that improves by the day and even the hour in active-use scenarios.
- ModernMT is context-sensitive.
- The ModernMT system manages context automatically and does not require building domain-specific systems.
- ModernMT is easy to use and rapidly scales across varying domains, data, and user scenarios.
- ModernMT has a data collection infrastructure that accelerates the process of filling the data gap between large web companies and the machine translation industry.
- Driven easily by the source sentence to be translated and optionally small amounts of contextual text or translation memory.
ModernMT’s goal is to deliver the quality of multiple custom engines by adapting to the provided context on the fly. This fluidity makes it much easier to manage on an ongoing basis as only a single engine is needed.
The translation process in ModernMT is quite different from common, non-adapting MT technologies. The models created with this tool do not merge all the parallel data into a single indistinguishable heap; separate containers for each data source are created instead and this is how it maintains the ability to adapt to hundreds of different contextual use scenarios instantly.
ModernMT consistently outperforms the big portals in MT quality comparisons done by independent third-party researchers, even on the static baseline versions of their systems.
ModernMT systems can easily outperform competitive systems once adaptation begins, and active corrective feedback immediately generates quality-improving momentum.
The following charts show how ModernMT is a consistent superior performer even as the quality measurement metrics change over multiple independent third-party evaluations conducted over the last three years. None of these metrics capture the ongoing and continuous improvements in output quality that is the daily experience of translators who work with dynamically improving ModernMT at Translated Srl.
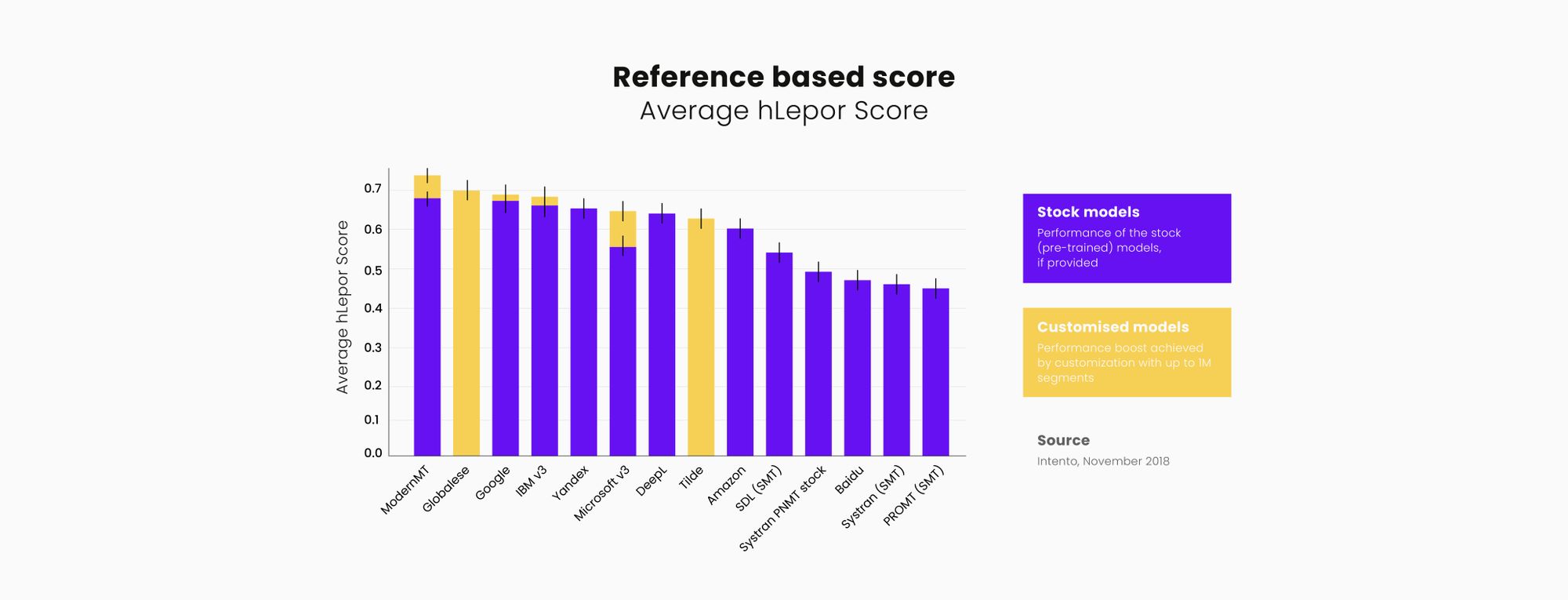
Independent evaluations confirm ModernMT quality improves faster with COVID data set on English > German. ModernMT was also the "top performer" on several other languages tested.
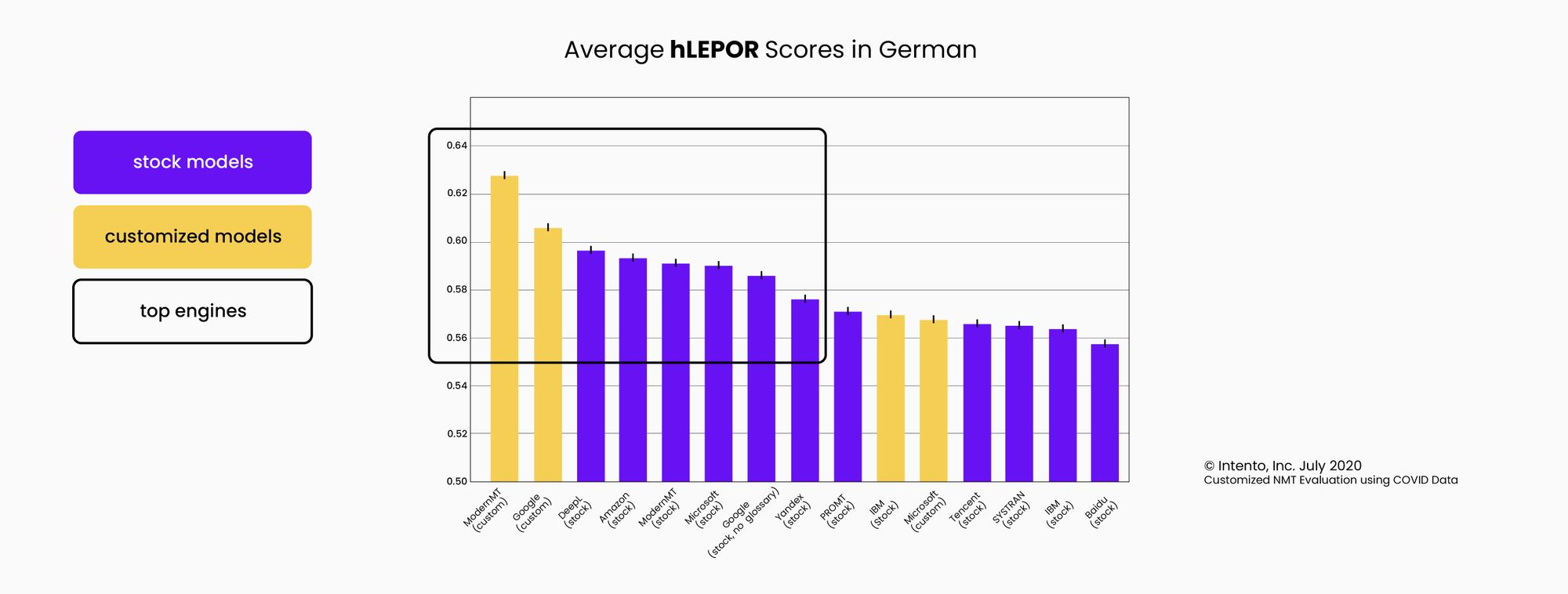
In Q4 2021 the COMET metric is widely being considered a "better" score because it is more aligned with human assessments and also incorporates semantic similarity.
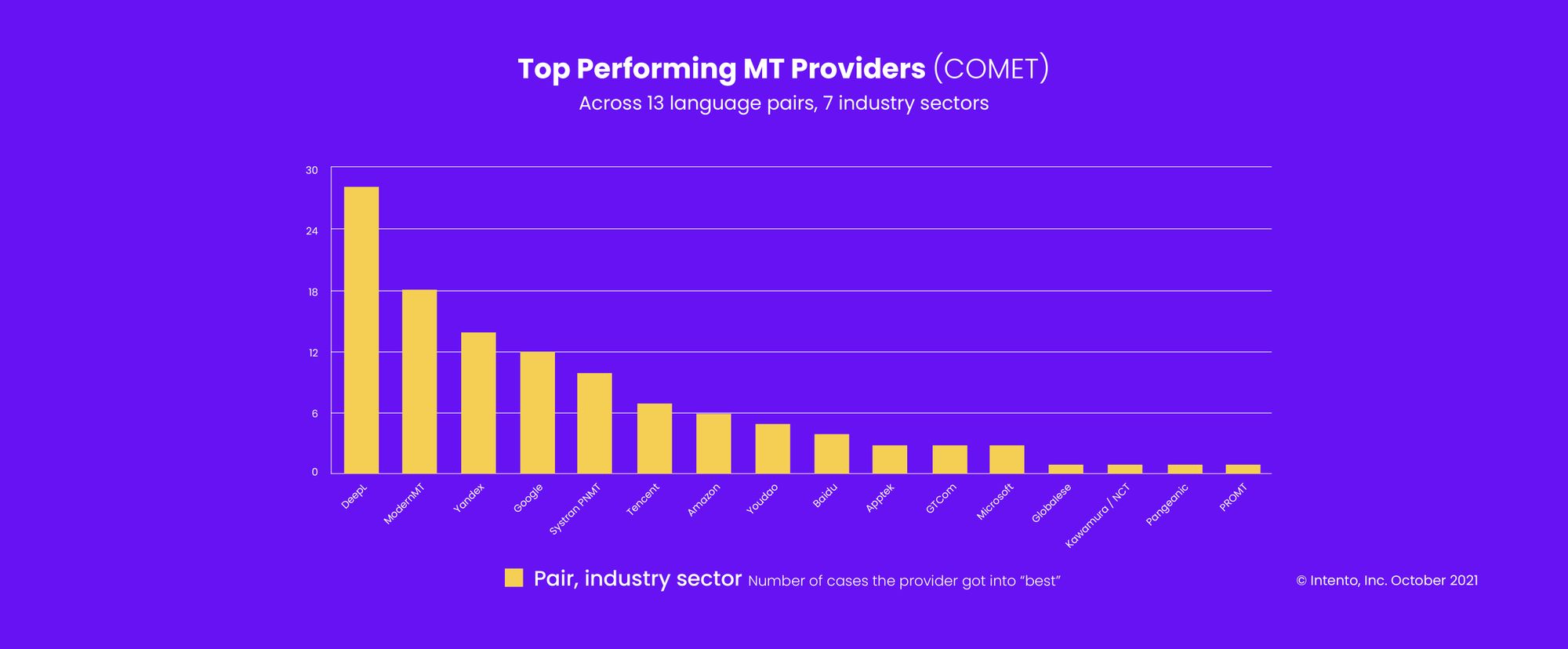
If the predictions about the transformative impact of the deep learning-driven revolution are true, DL will likely disrupt many industries including the translation industry. MT is a prime example of an opportunity lost by almost all the Top 20 LSPs.
While it is challenging to get MT working consistently in localization scenarios, ModernMT and Translated show that it is possible and that there are significant benefits when you do.
This success also shows that when you get MT properly working in professional translation work, you create competitive advantages that provide long-term business leverage. The future of business translation increasingly demands collaborative working models with human services tightly integrated with responsive, adapted MT. The future for LSPs that do not learn to use MT effectively will not be rosy.
A detailed overview of ModernMT is provided here. It is easy to test it against other competitive MT alternatives, as the rapid adaptation capabilities can be easily seen by working with MateCat/Trados or with a supported TMS product (MemoQ) if that is preferred.
ModernMT is an example of an MT system that can work for both the LSP and the Translator. The ease of the "instant start experience" with Matecat + ModernMT is striking when compared to the typical plodding, laborious MT customization process we see elsewhere today. Try it and see.
ModernMT is a product by Translated.